Rethinking AI: Stepping Back from the Generative Hype
Over the past few years, companies have struggled to find meaningful ways to engage with the AI transformation—and no wonder. The development of generative AI and large language models has been so rapid that it has been almost impossible for companies to keep up and to fully in-source the knowledge. But there are better ways to learn.

Open Invitation
Workshop for companies: Practical Value from Data and AI
23rd of September at 13:00-15:00
at VTT Future Hub (Maarintie 3, Espoo)
To reserve your seat, please send an e-mail to [email protected] by 19th of September.
Authors: Juri Mattila (VTT), Pasi Pussinen (VTT), Arash Hajikhani (VTT), Timo Seppälä (Aalto University),Heikki Ailisto (VTT)
Empty Barrels Make Loud Noises
In recent years, some very extravagant claims have been made about the future value of AI to companies. For example, in 2023, the economists at Goldman Sachs predicted that AI will eventually increase annual global GDP by 7 percent.[1]
In a more critical take, MIT’s economist Daron Acemoglu recently stated that the GDP growth from AI may only be about one percent over the next decade, in total. As Acemoglu astutely points out, it seems that the current mainstream trajectory of AI development, which places a lot of emphasis on large language models and foundation models, may not be the golden ticket that companies are looking for.[2]
Saying Isn’t Playing
To illustrate the problem, imagine we set up a chess match between two different AI systems. On one side, we would have IBM’s Deep Blue—the chess computer that beat Garri Kasparov, the reigning world champion at the time, back in the year 1997. The other side would be played by the latest and most developed flagship model of OpenAI’s ChatGPT. It may be tempting to think that with the rapid advancements of generative AI, ChatGPT would have a leg up on its adversary from a bygone era. But in a match like this, Deep Blue would almost certainly win, hands down.
As a generalized system, ChatGPT relies on inferring context and meaning from vast amounts of mainly textual data. It can convincingly discuss chess moves and chess strategy, just as it can very convincingly tell you which chess piece best describes each character in the Wizard of Oz. But attention to those kinds of associations is all it has to rely on. When something doesn’t fall in line with how things usually are connected, ChatGPT is completely lost in the woods.
Deep Blue, on the other hand, is a hardwired beast, an apex predator in its natural environment. Everything about its existence has been designed and built from the ground up to do one thing only: to systematically find the next best move for any particular layout on the chess board and to outsmart and destroy its opponent by sheer overwhelming brute force.
Despite the hundreds of billions of parameters of statistical dependencies, and terabytes of training data, a state-of-the-art large language model still couldn’t hold a candle to an old chess computer from almost 30 years ago when it actually comes to playing chess. While large language models can eloquently discuss the game, they still cannot compute it.
Quick Bloom from Deep Roots
Herein lies the issue that is so very easy to miss amidst all the AI hype. The recent developments in generative AI and large language models can make it seem like we are witnessing a rapid and sudden transformation of everything, almost comparable to the Industrial Revolution in the early 1800s. However, as a sobering thought, it’s important to remember that artificial intelligence is, in fact, by no means a novel phenomenon. The history of neural networks, for example, reaches back about 80 years. Likewise, the first industrial applications of machine vision were utilized almost a full century ago to sort food items by photoelectric cells in the 1930s.[3]
So, are generative AI and large language models disruptive in some capacity? Certainly. Do they make every other development in AI and data-driven business obsolete in their wake? Most definitely not.
While large language models can be very powerful in some generalized language-based problem-solving tasks, such as summarizing documents and drafting memos, they are simply not particularly well suited to the vast array of the real-world business problems and the value-generating needs that companies have. Developing large language models is costly, and often the pre-trained foundation models come with limited configurability. Industry applications also typically have limited amounts of data associated with them, so fine-tuning them with large training sets is not necessarily always feasible. Furthermore, if one truly dissects the conceptualized AI use cases down to the very basic level, it’s evident that most real-world problems that companies are faced with in their daily operations are simply not language-based problems to begin with, but well-defined problem domains based on pre-coded knowledge representation and reasoning, just like the game of chess.
Just as there would be no added benefit to Deep Blue from understanding the nuances of how Shakespeare’s works have shaped the history of English literature, for most tasks, large foundation models are simply way too overkill. In the coming years, some of the more recent avenues in AI research, such as transfer learning, in-context learning, and zero-shot learning, may provide useful in harnessing large language models for more practical value creation for businesses. However, for genuine value creation now and in the future, in the vast majority of cases, reliability is far more important than adaptability. Until a fundamental reorientation of focus takes place to that effect, the development of generative AI and LLMs may continue to leave companies holding an empty sack.
It’s Time to Challenge the Narrative
While generative AI gets all the headlines, there are better ways for companies to learn, with less risk and less cost—and with more immediate and more tangible benefits on the horizon.
For example, for many companies, the smart thing to do would be to start with the basics, and to focus on their data operations rather than complex models. If there is one thing that’s true with all of AI, it’s that if you put garbage in, you get garbage out. No complexity in the model can undo the damage from sufficiently bad inputs. Developing high quality data operations can provide much more tangible benefits, even when applied together with very basic AI tools, such as conventional machine learning.
Secondly, where attention to conceptual patterns is needed, companies should consider using transformer-based models that are tailored for specific tasks, rather than large, generalized language models. Simpler retrieval-based models can often perform better in more narrow business contexts. Moreover, in cases where language models are required, focusing on more specialized and task-specific small language models may be more effective and cost-efficient than trying to fine-tune large, general-purpose models like foundation models.
Furthermore, where language models are needed, it may be more helpful to put emphasis on small language models, or even symbolic AI, rather than trying to fine-tune large foundation models.
Call to Action
We are calling out to all the Finnish companies interested in developing their data and AI operations towards this more practical direction to join us in a workshop where we discuss our joint challenges, and discuss our plans to establish a research consortium with selected companies for a Business Finland Co-innovation project during this autumn in 2024.
We ask that you bring your own use case to be studied for the applicability of practical approaches to AI and data-driven business, focusing on tangible value creation and learning, not only on the technology, but also on the business side of the equation. Our intention in the project is to identify the business value drivers in these use cases related to data and high-reliability AI technologies such as machine learning and specialized transformers, to seek out the business value of these use cases, and to demonstrate some of those solutions together with companies. Join us in our journey to explore the horizons of practical value creation from data and AI.
Show other posts from this blog

Lampaita ja narreja vaiko kettuja ja supersankareita? Alusta palaa!
Vuosikymmeniä olemme ilmaiseksi luovuttaneet elämämme dataa teknologiayrityksille. Onko meistä tullut lampaita ja narreja internet-oven avauksella?
Suomen innovaatiorahoitus osa 3 – Tuotekehityksen jalostusarvo ja sen maantiede
Myös yritysten T&K-toiminnan tuotoksia ja tuottavuutta tulee mitata, toteavat Aino Salmi ja Timo Seppälä tuoreessa kolumnissaan.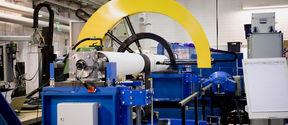
Haluatko pelastaa maailman ja tulla miljonääriksi? Katse omaan napapaitaan!
Jari A.T. Laine ja Timo Seppälä tarkastelevat kestävän kehityksen ympärillä tapahtuvan uuden teollisen kierron haasteita.