Creating the perfect teacher: How a cumbersome start with Japanese led to a better self-teaching app
Aurélien Nioche describes his recent work on a model that predicts learning outcomes days into the future

Aurélien Nioche, a postdoctoral researcher at the Aalto School of Electrical Engineering and the Finnish Center for Artificial Intelligence, recently published the paper Improving Artificial Teachers by Considering How People Learn and Forget with his coauthors.
What’s the exciting result from your paper?
This paper explores how to design better self-teaching applications, of the likes of the language-teaching Duolingo and Memrise. To do this, we have built a model that depicts what a user remembers about the learning material at a given time. With this so-called memory model - for which we only need a few interactions between the application and the user and no pre-existing data at all - we can predict what the user will learn several days into the future. We then use the model’s predictions to adapt the learning sequences to the user, and ultimately make learning with the application more efficient. From my perspective, the most exciting thing is that this model, despite being really quite simple, works well compared to the industrial standard.
How did the idea come about in the first place?
I was trying to learn Japanese by using self-teaching applications. I saw that most of the applications I was using were relying on a system that was not very flexible, with hard-coded rules. That’s why I started thinking: Could we develop an application that better adapts to its user by explicitly modeling what the user currently remembers? And here, the work began.
How is the paper different from what had been published before?
Outside of technicalities, the main difference to previous publications is our system (i) adapts to each user and each learning item, (ii) anticipates what will happen after several training sessions, and (iii) does all of this without relying on preexisting data.
Who did you collaborate with, and why?
Firstly, I collaborated with Pierre-Alexandre Murena, whose background is in machine learning. As my own background is in cognitive science, the collaboration was very fruitful for this project that combined both. I also collaborated with Carlos de la Torre-Ortiz who came to Aalto University originally as a summer intern in this project and is now doing his Ph.D. at the University of Helsinki. Carlos is very experienced in web technologies and handling server configurations, which was very helpful (configuring a server can sometimes be epic for the neophyte). The paper’s last author, Antti Oulasvirta, helped us never to forget the ‘big picture’ while we were struggling with technical issues. This way, we were sure that we were still solving the problem that we were originally focused on. Keeping on course is actually way more complicated than you’d think!
What’s the next paper you have planned? Will it be similar to this work or different?
We are currently working on two extensions.
First, we are developing a hierarchical version of the inference algorithm. The general idea is to benefit from the information that we can get at the population level to make better predictions at the user level. Don’t get me wrong; the core idea is still to adapt to each user and item. What we do is we begin by assuming that the user has an average behavior, and then, the more we know about the particular user, the more we can adapt to their specificities.
Secondly, we are developing a deep reinforcement technique that, based on a description of the user’s memory, learns the optimal strategy to meet personal teaching objectives. The general idea here is to use the data collected during this study to simulate human-like learners and use them for training the artificial teacher. The advantage of this technique is that it opens the possibility to further personalize the teaching by being more flexible about what the teaching objective can be. We’d like to be able to say: Give me what you want to achieve, and I’ll train the perfect teacher for you!
________________
After completing a Ph.D. in cognitive science at Sorbonne University (Paris, France), Aurélien Nioche is now a postdoctoral researcher at Aalto University in the User Interfaces group. By combining cognitive modeling and machine learning, his current work aims to understand how, in a teaching context, the collaboration between artificial intelligence (AI) and humans can be improved.
Show other posts from this blog
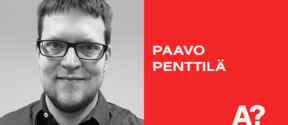
Röntgensäde vie puusoluseinän sisään
Puun mahdollisuudet uusiutuvana raaka-aineena ovat valtavat.